The Importance of Medical Image Annotation in Modern Healthcare
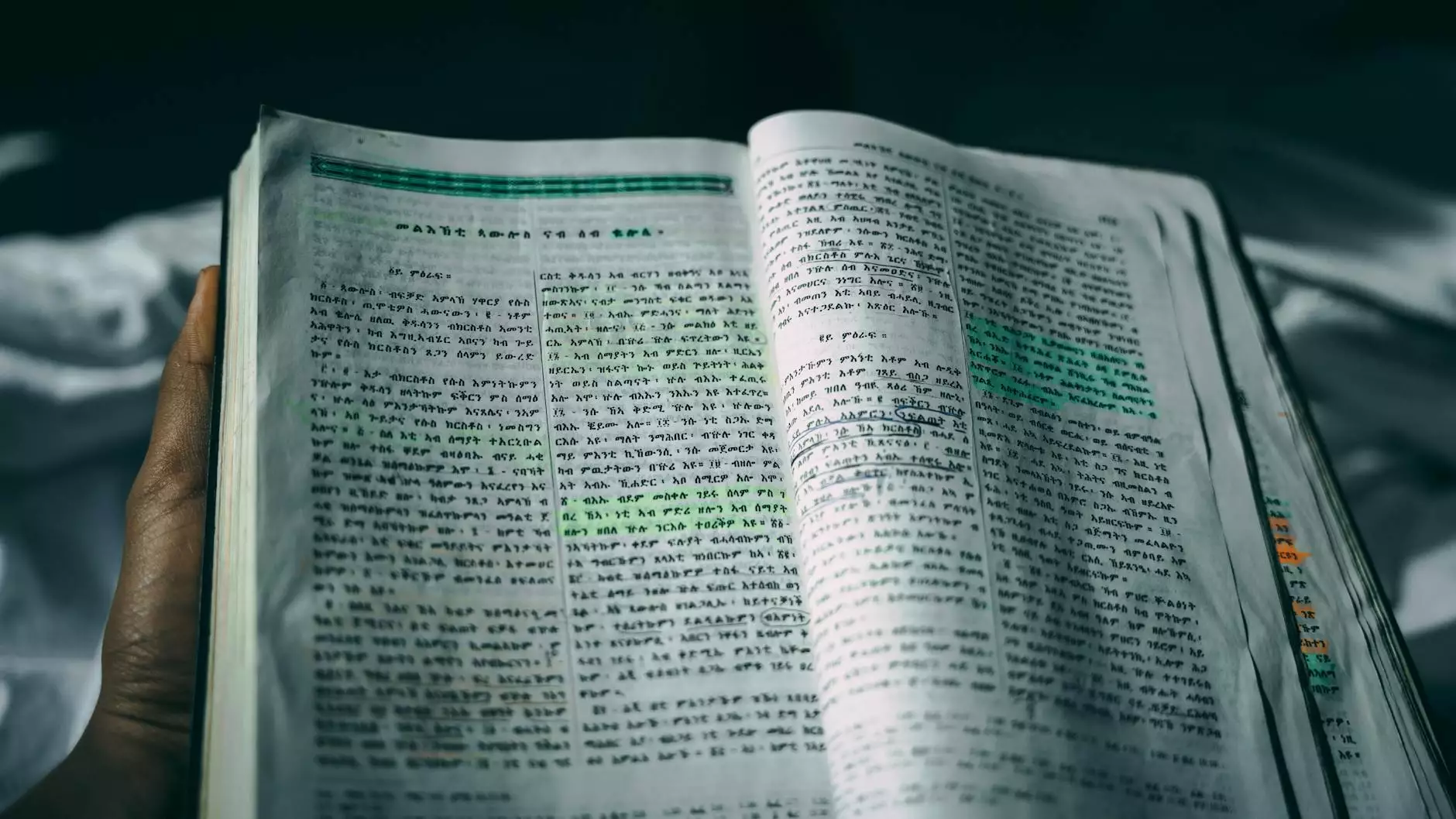
In recent years, the realm of healthcare technology has witnessed revolutionary changes, particularly through the integration of artificial intelligence (AI) and machine learning. Among these advancements, one of the most significant is medical image annotation, which promises to enhance the accuracy of diagnoses and improve patient outcomes. This comprehensive article delves into the multifaceted world of medical image annotation, illustrating its vital role in healthcare, its applications, and its future prospects.
What is Medical Image Annotation?
Medical image annotation refers to the process of labeling or tagging various features within medical images, such as X-rays, MRIs, CT scans, and ultrasounds. This tagging allows AI algorithms to learn from the images, enabling them to identify and quantify abnormalities in future scans automatically. In essence, the quality of the annotation directly affects the performance of the AI model and the reliability of its diagnostic insights.
The Process of Medical Image Annotation
The process typically involves several steps, which can be outlined as follows:
- Image Acquisition: High-resolution medical images are obtained from various imaging modalities.
- Preprocessing: Images may require preprocessing to enhance quality, reduce noise, and normalize lighting.
- Manual Annotation: Radiologists or trained technicians input various labels, marking areas of interest such as tumors, lesions, or anatomical landmarks.
- Verification: The annotated images undergo a rigorous verification process to ensure accuracy.
- Model Training: The annotated data sets are used to train AI models, enhancing their diagnostic capabilities.
Applications of Medical Image Annotation
The applications of medical image annotation are broad and have impacted various sectors within healthcare:
1. Enhanced Diagnostic Accuracy
One of the primary benefits of medical image annotation is its contribution to enhanced diagnostic accuracy. By providing AI tools with labeled training data, healthcare providers can achieve more consistent and reliable diagnoses.
2. Disease Detection and Monitoring
Medical image annotation plays a crucial role in the early detection and ongoing monitoring of diseases, including cancer and cardiovascular conditions. By accurately identifying tumors, radiologists can make timely decisions regarding treatment options.
3. Research and Development
In the realm of medical research, annotated medical images are imperative for developing new therapeutic protocols and understanding disease progression. Researchers utilize these data sets to identify trends and develop predictive models.
4. Personalized Medicine
With the aid of accurate medical image annotation, healthcare professionals can tailor treatment plans that cater specifically to the unique characteristics of each patient's condition, ushering in a new era of personalized medicine.
Key Technologies Driving Medical Image Annotation
The following technologies are essential in the efficiency and effectiveness of medical image annotation:
- Artificial Intelligence and Machine Learning: Cutting-edge algorithms analyze vast amounts of imaging data, learning from annotated images to improve diagnostic precision.
- Deep Learning: Deep learning models, such as convolutional neural networks (CNNs), excel at feature extraction and classification within medical images.
- Cloud Computing: Cloud platforms allow healthcare institutions to manage massive datasets securely and effectively, facilitating collaborative annotation and training efforts.
- Open-source Tools: Several open-source software solutions have emerged, enabling more organizations to engage in image annotation without significant financial barriers.
Challenges in Medical Image Annotation
Despite its benefits, medical image annotation does face several challenges:
1. Annotator Variability
Diagnosis can be subjective. Different annotators might label the same image differently, leading to inconsistencies in the training data. Standardizing annotation protocols is essential to mitigate this issue.
2. Scalability
As the volume of medical images increases, so does the demand for annotated data. Scalability becomes a major challenge for healthcare facilities with limited resources for manual annotation.
3. Data Privacy Concerns
Given the sensitive nature of medical data, strict adherence to regulations like HIPAA (Health Insurance Portability and Accountability Act) is necessary to protect patient privacy during the annotation process.
The Future of Medical Image Annotation
The future of medical image annotation is promising, driven by advancements in technology and increasing demand for diagnostic accuracy. Here are some trends expected to influence the field:
1. AI-Powered Annotation Tools
Future annotation tools are likely to leverage AI to automate parts of the annotation process, significantly increasing efficiency while maintaining high accuracy rates.
2. Integration with Augmented Reality (AR)
Augmented reality may enhance the visibility of a patient’s anatomy during diagnoses and help in the training of medical professionals through interactive annotations.
3. Real-time Annotation
As technology develops, the capability for real-time annotation during imaging procedures is anticipated, allowing for immediate decision-making.
4. Increased Collaboration Among Institutions
Healthcare institutions may start collaborating more extensively, sharing annotated datasets to build more robust AI models and improve collective diagnostic skills.
Conclusion
In summary, medical image annotation stands at the forefront of technological advancements within healthcare, reshaping how diagnoses are made and medical treatments are delivered. By harnessing the power of AI and structured annotation processes, healthcare professionals can unlock new potentials, paving the way for improved patient outcomes and innovations in medical research. As we look ahead, the sustained development and investment in medical image annotation technologies will be vital in overcoming existing challenges and capitalizing on opportunities to enhance patient care.
At KeyMakr, we specialize in cutting-edge software development solutions that empower healthcare organizations to leverage medical image annotation effectively, ultimately striving to improve diagnosis and treatment through innovative technology. Our commitment is to provide tools and services that meet the highest standards of accuracy, performance, and compliance with health regulations.